Feature Importance Networks
There exists within computational neuroscience, among a number of other fields, a significant ideological gap between descriptive statistics and machine learning or classification based approaches. The general trade off in approaching problems of interest within a machine learning framework, i.e., complicated classifiers, is a boost to predictive performance at the cost of interpretability. On the other hand, the more traditional descriptive statistic approaches concern themselves for the most part with attempting to explain what is going on, and therefore by design yield only results under a certain threshold of complexity. Within the scope of this work I attempt to further bridge the gap between complexity and explain-ability via the construction of a feature importance network.
See the full project write-up here
This was a course project, and my final presentation can be found below.
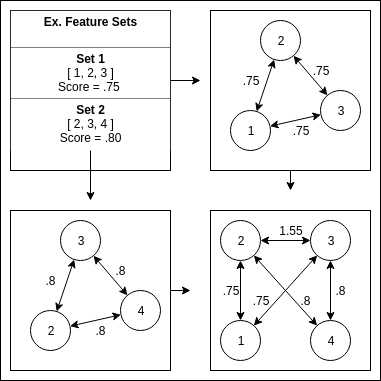
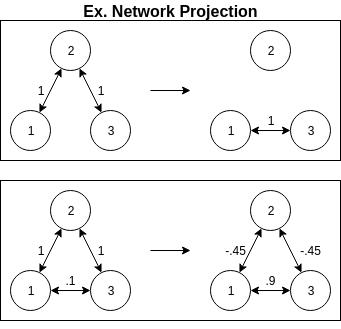